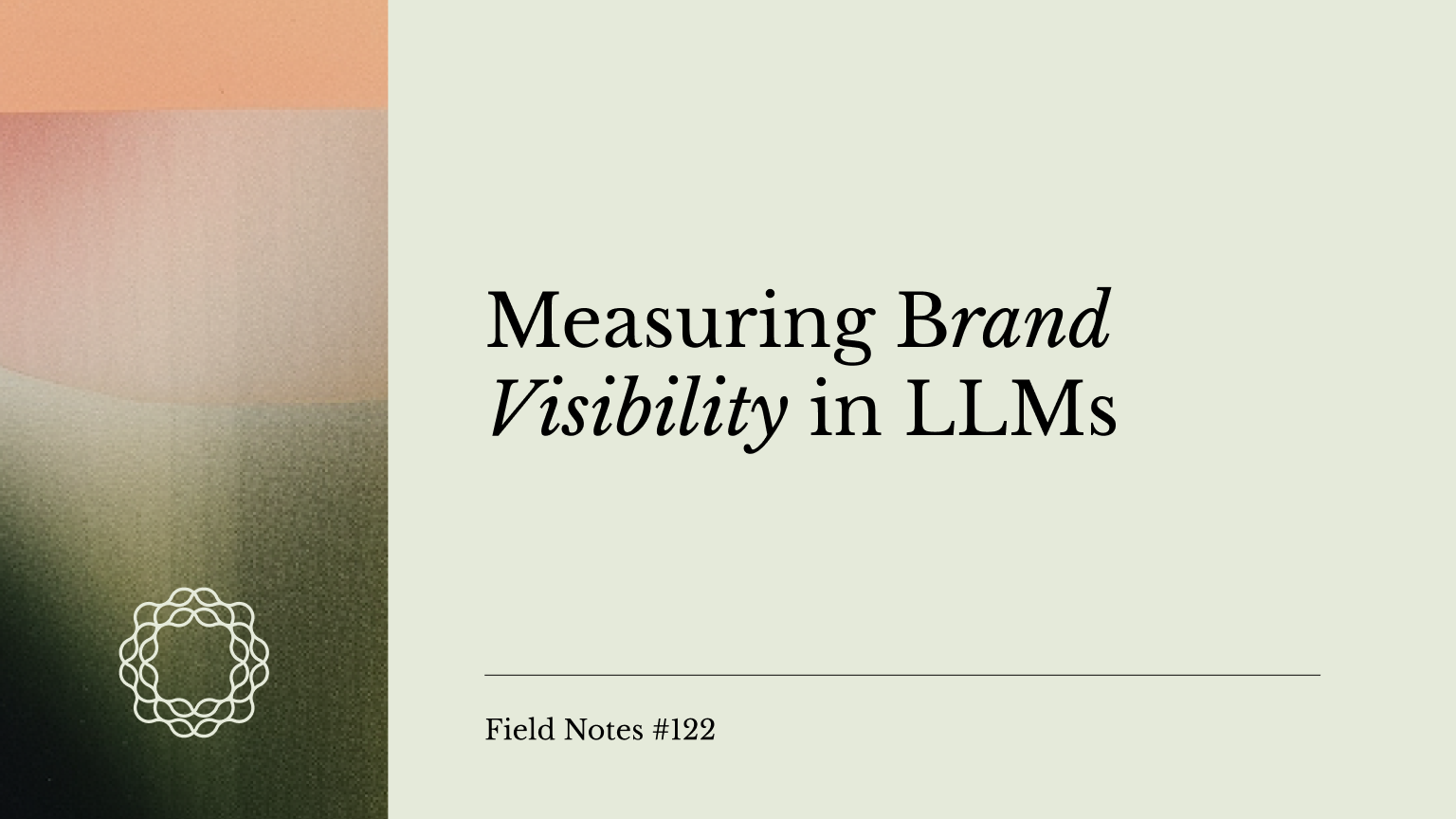
There’s a bit of beauty in classic SEO measurement frameworks, especially when you abstract them from revenue and business outcomes (which I do not advise).
Traffic is easy to track.
In Google Analytics, it automatically buckets traffic by source and page, so you can easily parse out organic from paid, and blog posts from product pages.
In a world of ten blue links, you can assume position 1 is superior to position 7, even run some click-through-rate curves, measuring visibility, performance, and opportunity from the SERP level.
Ignoring that this, too, was always imperfect, it was, at the very least, scrutable.
You could easily show charts to executives and one could comprehend, with little knowledge of SEO, that charts going up and to the right were largely a good thing.
AI kind of breaks that down.
Well, it furthers a trend that has been happening across other platforms, that which we can largely call “dark traffic,” as you surely know of dark social.
Basically, more information consumption and decision making is occurring in opaque areas like communities, WhatsApp groups, social (with no click), and now, by using AI tools, agents, and simply by accessing information that does not require a click. We’ll also likely see more agents interfacing with your content and brand, which as of now, are hard to distinguish from human users. A murky and confusing, but exciting, new frontier.
If you assume that these tools will a) get better and b) rise in usage, then it’s pretty apparent that they are and will continue to be an important surface area for organic marketing efforts.
And I do believe we should try our very best to measure what we can (and to accept what we cannot measure, channeling our inner stoic).
What’s changing?
Quickly, here’s how I see the difference as it stands today between SEO and GEO (I really hate that acronym, but you get it) measurement.
The Old Model:
- Keyword rankings
- Impressions
- Click-through-rate
- Organic traffic
- Linear attribution models (first/last click)
The New Model:
- Infinite prompt variability, little volume data available
- Questions / conversation > keywords
- “Rankings” don’t really translate
- Visibility varies and often includes multiple prompts and conversations
- Clicks (maybe)
- Information largely consumed within platform with some referral traffic available from links and citations (differs on platform)
- Higher intent
- Despite all of that, leads from AI tools seem to be much higher intent on average. This could be due to early adopter maturity, increased information parity before hitting demo / signup stage, or something else. But this traffic is good traffic.
I’ll get into how to measure this, as simply as possible, below. But first, I’d like to explain that this is not just a measurement problem and shift, but an alteration in the customer journey and how consumers interface with information and brands.
It’s not just the map, but the terrain, that is changing
Discovery is happening in spaces where traditional telemetry fails to penetrate.
That’s not a new thing, obviously. Dark social, word-of-mouth, brand…none of these are well measured by digital attribution systems.
Even in search, we’ve been dealing with an increasing number of zero click searches for years due to featured snippets and other SERP UI changes.
However, generative AI does work quite differently than traditional search (though they seem to be converging in interesting ways…)
AI tools do not simply retrieve documents in response to static keywords (I mean, Google search doesn’t really operate that way anymore either). They generate new outputs based on context, on memory, on personalization, and on an ever-shifting understanding of the world.
And with that change comes a disorienting reality for many SEOs: keywords are no longer stable units of measure, at least in the relatively linear way they used to be. They are being replaced by prompts, infinitely variable, deeply personal, often ephemeral.
This is a tectonic shift, more so from a consumer behavior standpoint than a strategic one, though it’s a shift that many SEOs will struggle to adapt to.
We’ve been advising for a while to base strategies on customer and buyer research, building out topical relevance through qualitative insights like voice of customer and pain point phrases, and mapping it to static dimensions like ICP qualifiers.
Still, we have to admit: keywords are easier to track.
Oh well, the world doesn’t mold itself around our rulers; our measurement systems must adapt to the way the world works.
Models help us make decisions, improve outcomes
In the face of such ambiguity, many marketers retreat into cynicism.
“You can’t track anything anymore,” they say. “Attribution is broken. What’s the point?”
But this is a misunderstanding, both of the nature of models and of the nature of strategy itself.
The purpose of a measurement model has never been to describe reality with perfect precision. Its purpose is to describe reality well enough to guide action. As the statistician George Box wrote (and has since been quoted to death by myself and others), “All models are wrong, but some are useful.”
What we need now is not abandonment of models entirely.
What we need are new models, ones that acknowledge the opacity of AI discovery, but that still offer us a structured way of seeing, deciding, and acting.
And I would argue we already have the contours of such a model.
So what do we do?
Simple (but not easy): Build a measurement model that’s useful — not perfect.
The Framework:
1. Lagging Indicators
- Actual pipeline: leads, users, revenue, from AI sources
- Self-reported attribution: “How did you find us?”
- Blended CRM and analytics data to form a richer picture of how AI plays into discovery and the customer journey today
2. Leading Indicators
- Referral traffic from AI tools (set this up in GA4 or similar)
- Visibility within AI tools (via Peec, Profound, Scrunch, Otterly, etc.)
3. Input Layer Visibility
- Brand mentions (especially on Reddit, Quora, 3rd party lists)
- Surround Sound SEO strategy to flood key SERPs and AI answer layers
- Topic / question coverage
A simple framework for AI measurement
It begins, like all good models, by grounding us in what is still measurable.
Lagging indicators
Regardless of what AI changes, revenue remains legible.
We can, and should, continue to track:
- Incremental pipeline sourced from AI discovery channels.
- Leads and users who self-report having found us through AI tools.
- Closed-won deals that emerged from journeys which, while perhaps murky, leave traces in the form of customer interviews and sales notes.
This is, in many ways, a return to fundamentals: ask your customers where they found you. Add a self-reported attribution field to every form. Probe in sales calls. It’s more important than ever, in my opinion. I also always ask follow ups on discovery calls, such as the specific prompts and journeys someone took within, say, ChatGPT, to get to us.
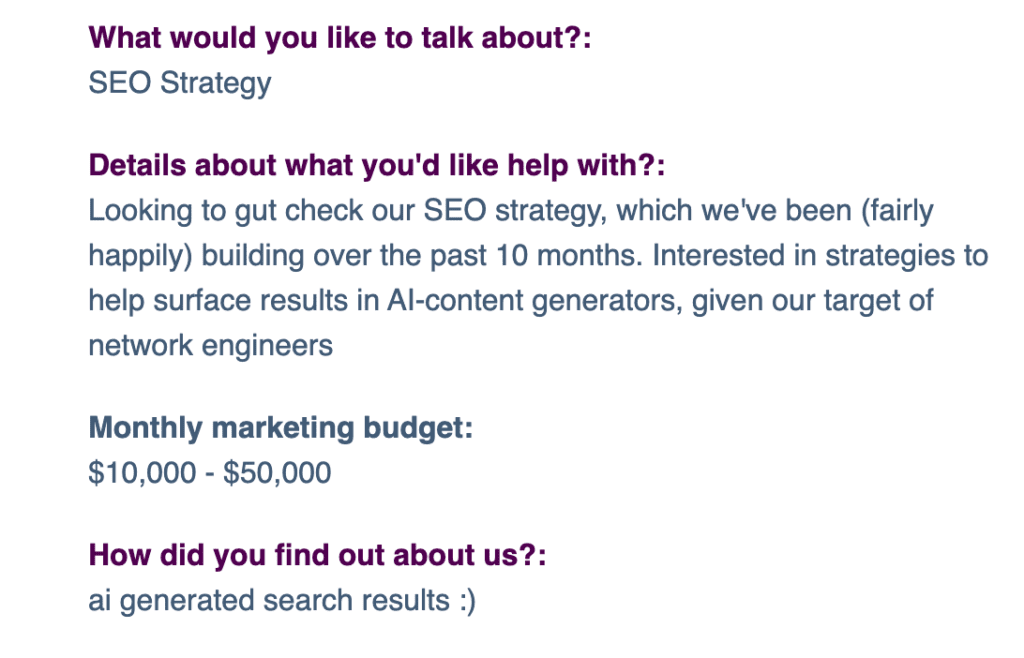
Leading indicators
While lagging indicators show outcomes, we still need signals for directionality. Leading indicators typically also represent data points that can help fuel better decision making on the input layer, whereas lagging indicators are often, well, lagging and take a lot of time to move.
This is where referral traffic from AI tools becomes valuable. Chat.openai.com. Perplexity, You.com. These domains are now among the most fascinating leading indicators a marketer can track.
We set up dashboards in Looker Studio that include AI engine filters, cut in various ways, to show aggregated traffic referrals, page by page data, and conversions resulting from these engines.
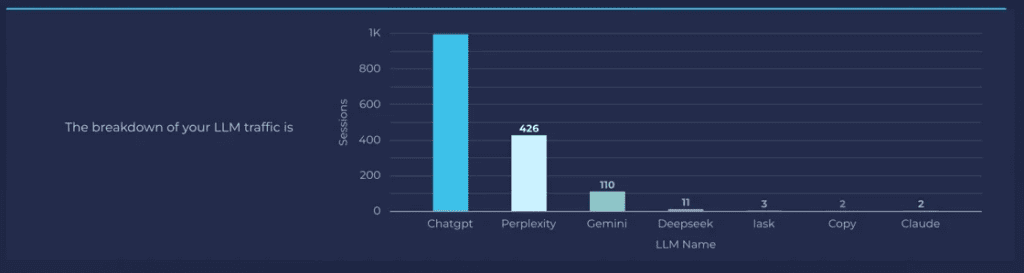
I take the conversion data here with a grain of salt for the aforementioned reason that a lot of the interaction in these systems happens away from your website tracking and cookies. Still, it’s interesting to see where key events are happening.
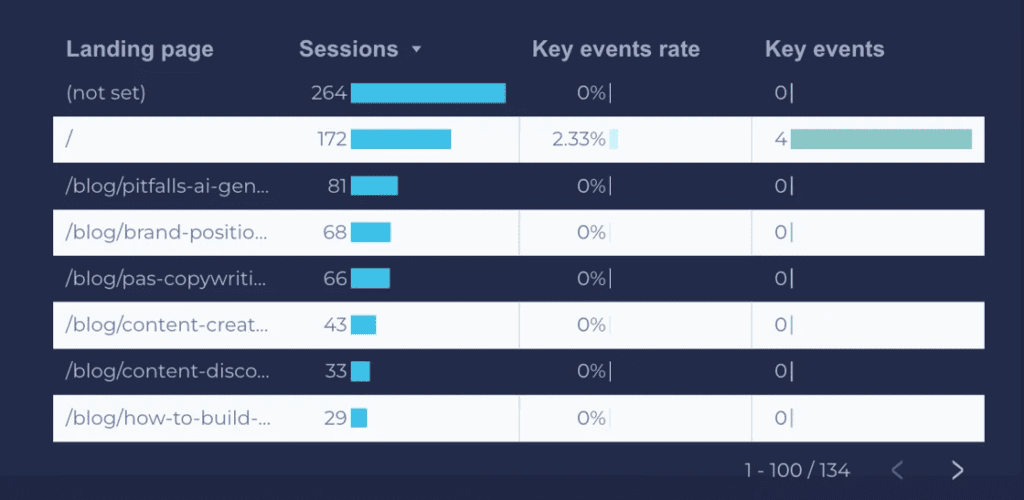
Visibility tools like Peec.ai, Profound, Scrunch, and Otterly (and you just know there are many more to come) offer imperfect but helpful glimpses into where your brand appears within AI-generated outputs. They often offer suggested prompts, frequency of citations appearing for the prompts, competitors and their stature in the same prompts, and your growth or change in relation to a set of prompts or in a specific AI tool.
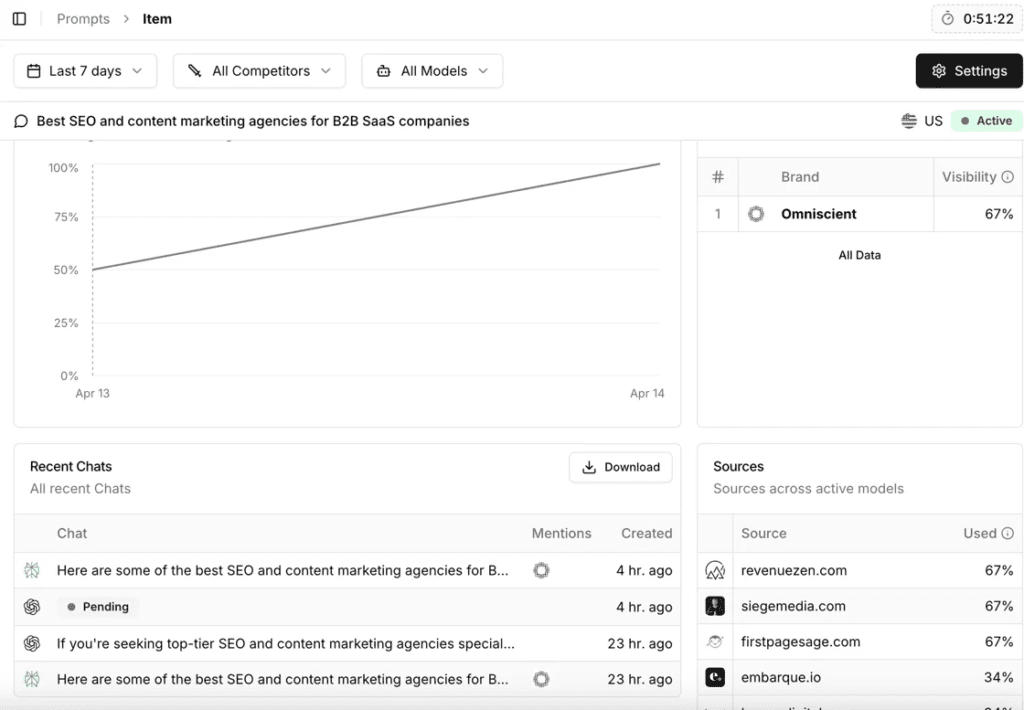
I expect these tools to get clearer and better in the future, but even now, they’re great for identifying opportunities in relation to your topic set (though you have to get out of the “keyword” mindset), and for identifying the most powerful sources and citations so you can work partnerships and Surround Sound SEO tactics.
Input layer saturation
Finally, there is the input layer, which is still based on a mixture of first principles, conjecture, and some very early data and pattern recognition.
The two key vectors seem to be answering ICP questions and conversational queries in specific, credible, and expert-backed ways (ideal if you can include original data), and increasing your brand mentions, especially in key citations. So basically great content strategy and digital PR / brand, not too different from the old days really.
On the margins, today at least, Reddit, Quora, and YouTube seem to be overrepresented in citations, so it may warrant distinct tracking for brand visibility in mentions in these places. Otherwise, topic maps and coverage should be common practice, and Surround Sound SEO auditing for your core category and BOFU terms will get you a long way. Look into:
- Managing visibility across Reddit, Quora, product listicles, review sites.
- Saturating not just SERPs, but the full landscape of public web content where AI tools derive knowledge.
- Focusing on Surround Sound SEO, especially for categorical keywords with existing demand and agreed upon nomenclature.
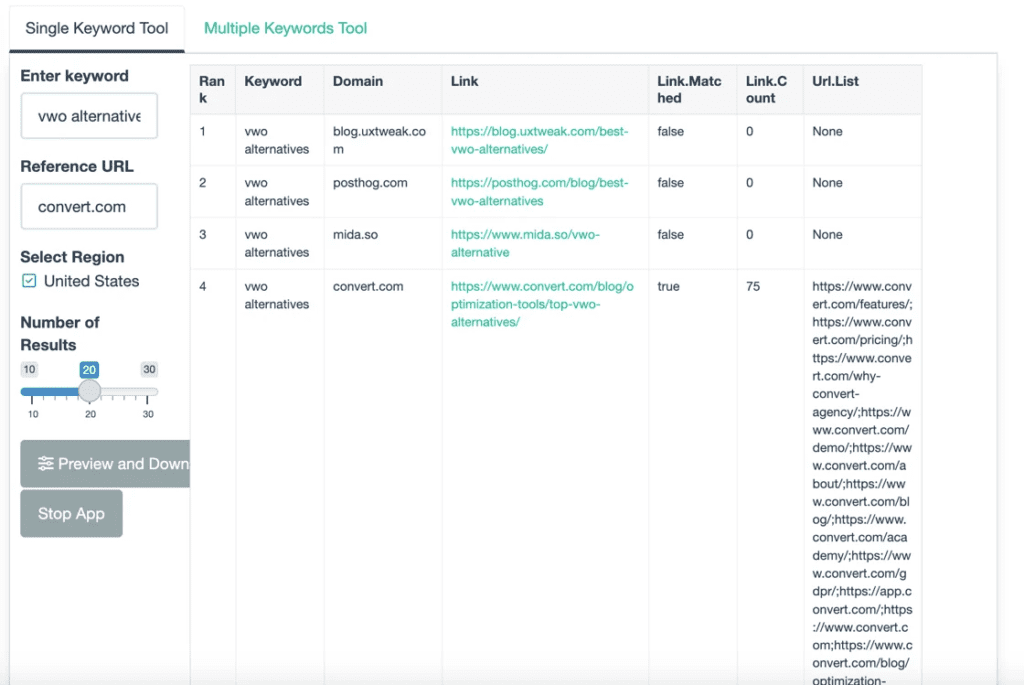
An internal tool designed to audit brand visibility and real estate for BOFU SERPs
Triangulation as the new epistemology
Taken together, this model does not restore the clarity of the past. It replaces linearity with triangulation, and at no point will you get near the Elysian ideal of perfect data (and one must courageously accept that and act anyway).
You look at incremental pipeline. You layer in referral traffic from AI tools, especially to stack rank opportunities. You examine visibility in the answer layer. You track self-reported attribution. You map brand mentions across influential surfaces. All of this in addition to classic analytics and attribution systems, which, together, will give you a clearer picture of where you stand, where you can improve or optimize, and some hypotheses to help you move the needle where needed.
Want more insights like this? Subscribe to our Field Notes.