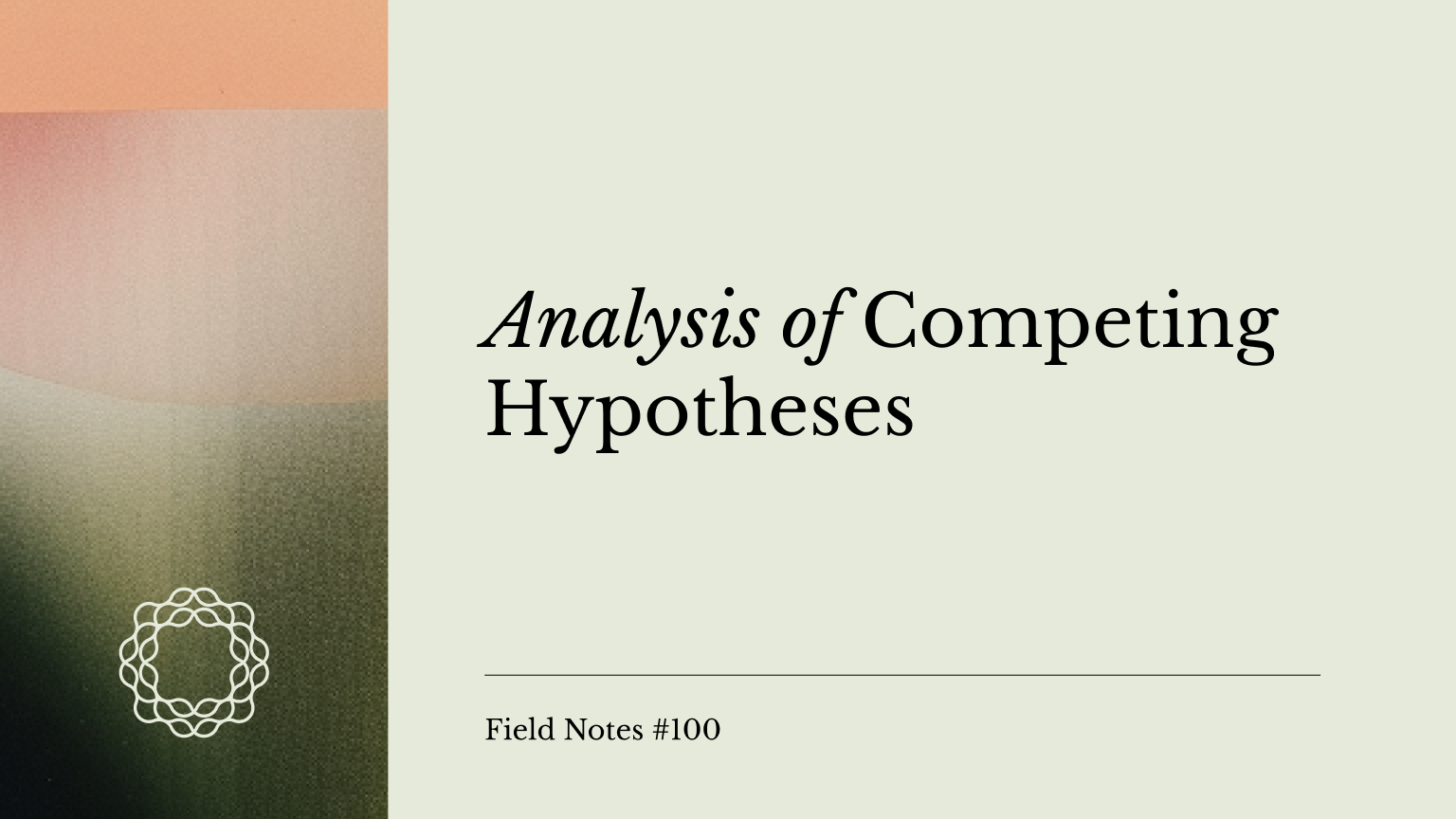
First a definition, and then I’ll get quickly into our story.
Analysis of competing hypotheses¹ (ACH) is a “process whereby you identify a set of hypotheses, systematically evaluate data that is consistent and inconsistent with each hypothesis, and reject the hypotheses that contain too much inconsistent data.”
I believe it was developed in the 1970s by the CIA, but we can use it for organic growth marketing, too.
Finding Root Causes with ACH: A Case Study
Recently, a client’s organic traffic had taken a noticeable dip over the past three months—nothing extreme, but enough to need answers.
Paradoxically, we noted positive trends among several content cohorts, including and especially with the new posts we had published for them. All were ranking well and driving steady visitor growth. But across the website, and particularly on the blog as a whole, traffic was nominally down month over month.
Time to investigate.
Our organic growth strategist, Usman, started by laying out all the possible causes, which is the first key step in ACH.
Every plausible explanation had to be on the table before we could eliminate anything.
- Was it a drop in branded (versus non-branded) traffic issue?
- A drop in keyword rankings?
- Competitive saturation?
- Reduced click-through rates in key SERPs?
- Seasonality?
- Or maybe even traffic “stolen” by AI overviews?
Each option carried weight, so Usman assigned a score to each one based on available evidence. It was a methodical process—no theory held unless it could withstand a test.
Through this process of elimination, the real cause started to emerge.
Around 70% of the traffic dip was coming from just four blog posts.
Next, we analyzed the granular keyword data for those posts and found a potential root cause: a significant, sustained drop in search volume for the core terms those pages ranked for, right in line with our timeline.
There it was: search demand had shifted, despite us holding on to our rankings (all in position 1-3 with no movements). We didn’t have to tweak pages, rework our internal links, or look at competitor gains. The demand wasn’t there; it had dropped.
With this insight, the path forward became somewhat less murky.
Knowing the source of the dip saved us from wasting resources on ineffective fixes. Instead, we could pivot and identify tactics that would incrementally move us forward.
With this demand drop verified, we now had options: we could shift to new topics where search interest was growing or invest in additional distribution channels for these pages.
And crucially, we could skip tactics like on-page SEO, technical SEO, or link-building for these specific posts—nothing we could add would create demand where it had faded.
Why ACH Works: Via Negative & Evidence Weights
ACH isn’t just about diagnosing traffic. At its core, it’s about stripping away noise, so you’re only left with the most feasible causes.
This mindset keeps you from clinging to favored theories or easy explanations, and it’s why ACH can be so powerful. Most remarkably, it’s a wonderful way to strip away potential biases from your analysis. As Moe Kiss puts it:
“Why do I love it? Often we set out to prove something and then are persuaded by certain key pieces of evidence until we confirm what we believed in the first place (aka confirmation bias).
ACH is the opposite — you set out to disprove something. The strength of this approach is that it is difficult to allow your natural biases to influence the outcome of your analysis. Particularly, in cases where you have missing information.”
Instead of collecting data for your preferred theory, we instead start from the via negativa model – a subtractive process that looks to disprove theories.
It’s essentially a form of root cause analysis, a method you might already know for narrowing down data trends to their origins. Here’s a deeper dive into root cause analysis if you’re interested in adding more RCA techniques to your toolkit.
In essence, by using this framework, we’re hoping to parse out the signal from the noise so we can focus more of our attention on the signal.
When working in experimentation, we had a similar concept known as “existing testing,” or in some circles as “conversion signal mapping.”
Before jumping in and optimizing a given UI element – say a welcome model upon entering a free trial for an app – you first determine how much that element matters in the first place (especially in relation to any other element).
How? Remove it. See what effect size it has. If removing it has zero impact, it’s unlikely tweaking the copy or design is going to have a very strong signal.
All of these tools are essentially ways of determining what matters and what doesn’t so you can spend more time moving the needle on what matters (and maybe ignoring the thousand tiny fires that an SEO tool will tell you are critical when you run an audit).
Learn More: A Practical Guide to Implementing ACH
Want to get more practical? Check out this detailed guide from Moe Kiss on implementing ACH for your own work.
In brief:
- Generate Hypotheses: List every plausible explanation for what’s happening in your data.
- Assign Evidence: Support or disprove each hypothesis based on available data.
- Rate Impact: Prioritize hypotheses based on which evidence has the greatest impact.
- Eliminate Hypotheses: Systematically eliminate what doesn’t fit, leaving only the most viable explanation.
- Take Action: Zero in on the best-supported hypothesis and use it to guide your next steps.
I’m no academic nor a CIA agent, so I don’t necessarily know the proper or formal way to lay this out. But that’s okay, because a) we’re doing SEO and not national security work and b) it’s more interesting to me that a tool is useful versus perfectly formal.
So you can format your tables however is useful for you.
In some cases (major strategic decisions, etc.), it’s warranted to run through this process at length and spend significant time on every bit of supporting evidence:
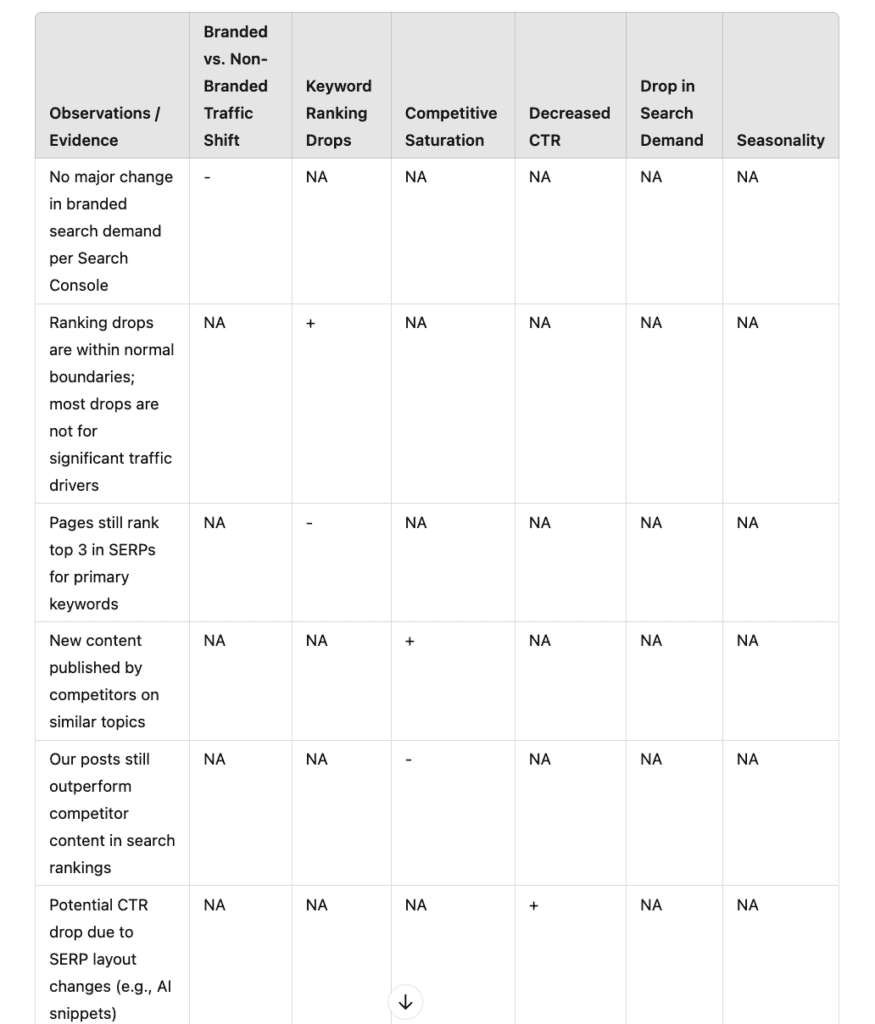
In most SEO cases, we can simplify and streamline the approach to excluding what is obvious to exclude and then applying what data we have to the emergent options:
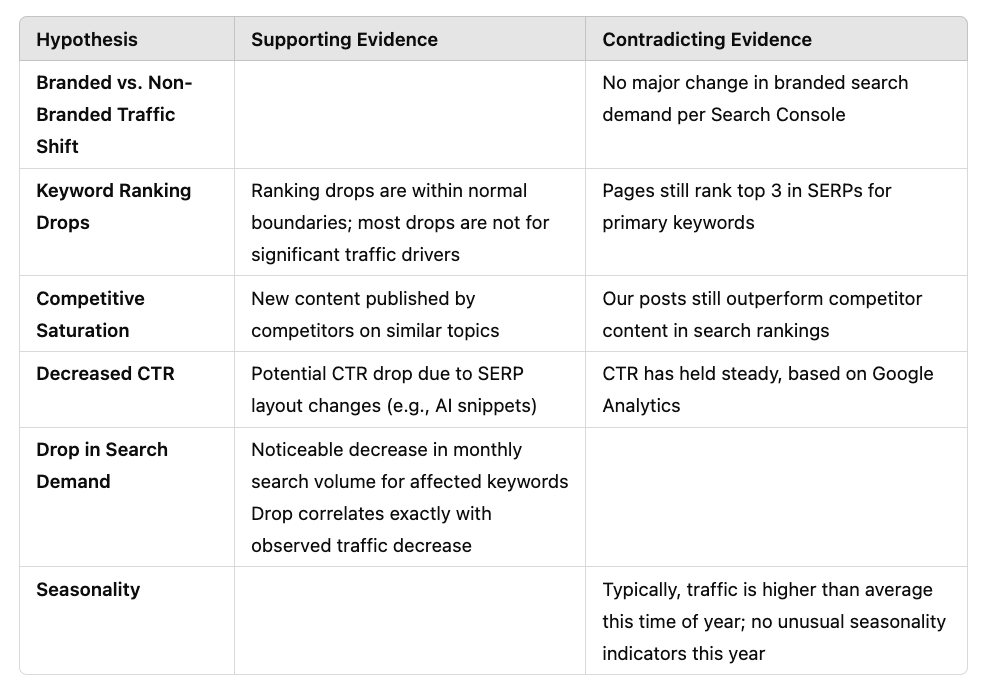
And in some cases, you don’t have to run this process at all. That’s what’s cool: you get to pick the tools you use to get to the desired outcome and better decision making.
There you go! A simple tool and framework to get a little bit more analytical, and a little less dogmatic and biased, in your SEO and growth work.
Want more insights like this? Subscribe to our Field Notes.