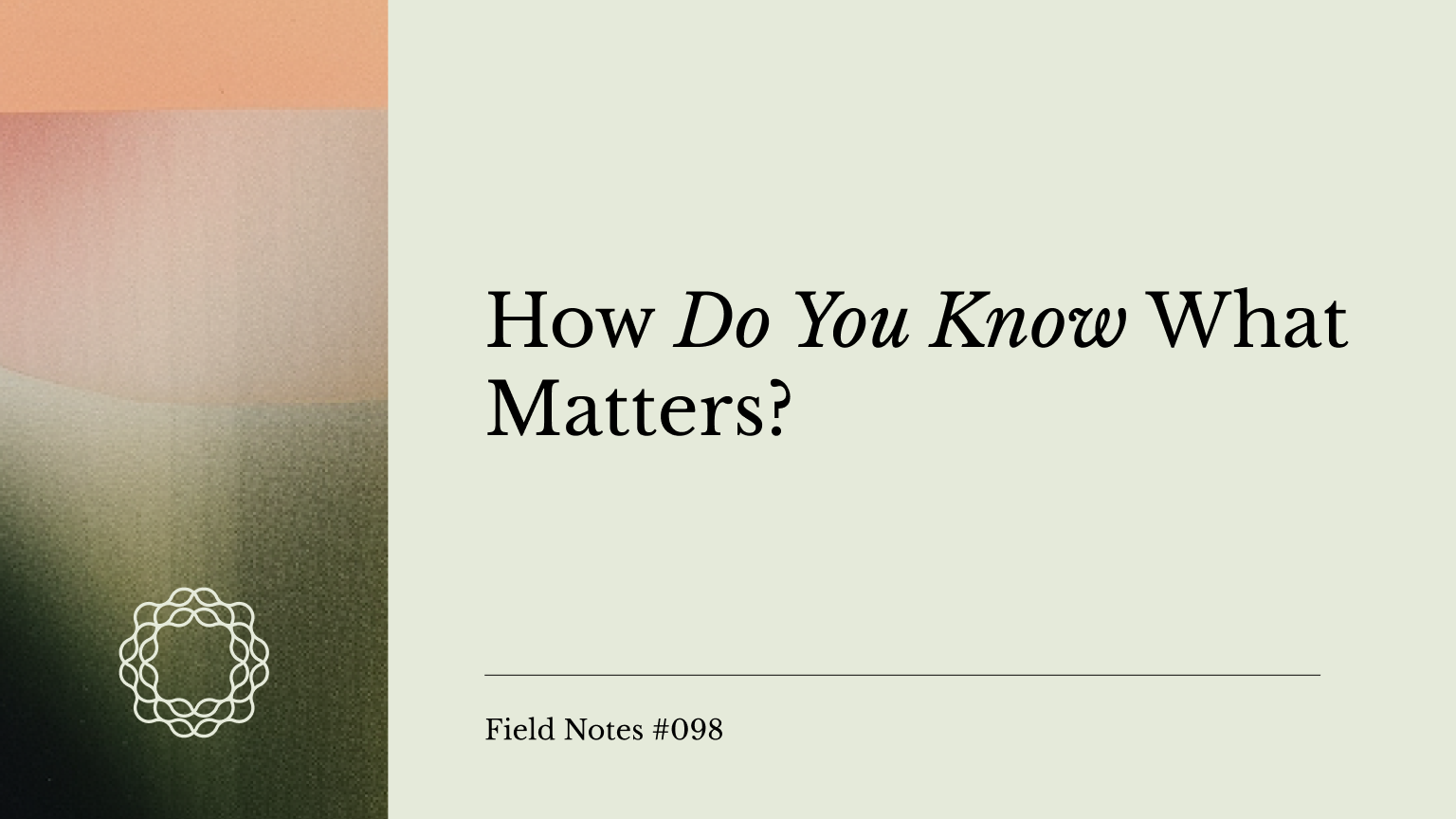
In some sense, this essay is exploring epistemological questions, like how we know what is true, how much evidence we need to believe in something, and how much evidence we need to make decisions.
However, it’s also tangible and maps back to questions I get all the time:
- What is the ROI of fixing N technical issues on our website?
- Can we quantify the impact of link-building on our rankings and traffic?
- What on-page SEO factors actually correlate with and predict better business outcomes?
- Should we increase content production, focus on optimization, increase/decrease links? I.e. what should we be focusing on as we scale to new growth horizons?
I’ll admit, I’ve said it before: SEO is simple (content, links, technical).
But it’s also a deeply complex system of interactions between publishers and their competition, users and their evolving behavior, and an intermediary directory with an evolving set of algorithms, machine learning, personalization, and AI features.
What we want to achieve is straightforward: we want to be visible in SERPs to connect with our target customers and convince them to consider our product or solution.
To do that, we must predict and approximate many factors (probably an endless amount), from who is searching for a given keyword, how many people, if that query is growing in popularity or shrinking, how we can best position ourselves to answer that qwerty, which factors Google (and now Perplexity and ChatGPT) deem most important to award us with that visibility.
It’s a complex system, but paradoxically, if we get bogged down in the complexity, we risk missing the forest for the millions of potential trees.
You don’t need to understand cell biology to eat well
Let’s look at a familiar example: nutrition science.
We’ve got mechanistic knowledge—understanding how certain nutrients should work in the body. But what happens when we study them in the real world? The body’s complexity, with its checks and balances, interaction effects, and unknowns, makes it hard to translate this knowledge into measurable outcomes. Still, we lean on correlations from observational studies and randomized controlled trials (RCTs) to make decisions. We also have cultural wisdom from our grandmothers that is usually on point.
Every few years a supplement seems to come out promising longevity (notoriously difficult to study in humans) because of its mechanistic and theoretical application. Resveratrol comes to mind. Lots of promise, but little evidence when it comes to human data or randomized controlled trials.
As they say, “In theory, theory and practice are the same. In practice, they are not.”
In SEO, we face similar challenges. Take backlinks, for example. The original Google patent emphasized the quantity and quality of links to a site, but how do you measure the causal impact of a single backlink today? How much does one link actually contribute to your rankings, and how much of it is just background noise in the larger system?
We know some things work because we’ve seen patterns over time (think of these as our observational studies), and we’ve got some micro-optimizations we can test directly (like A/B tests on title tags). But even with all this, we often rely on faith in the system—understanding mechanistically how backlinks or technical SEO should factor into rankings, but lacking precise numbers to back up each action.
So, how do you know what matters?
The hierarchy of evidence
In both science and SEO, not all evidence is created equal.
When you’re making decisions—especially in areas where the exact causal impact is hard to measure—you need to evaluate the strength of your evidence.
This is where the hierarchy of evidence, which I learned about from Ronny Kohavi, comes in.
It’s a pyramid that ranks types of evidence based on their reliability, from the strongest forms at the top to the weakest at the bottom.
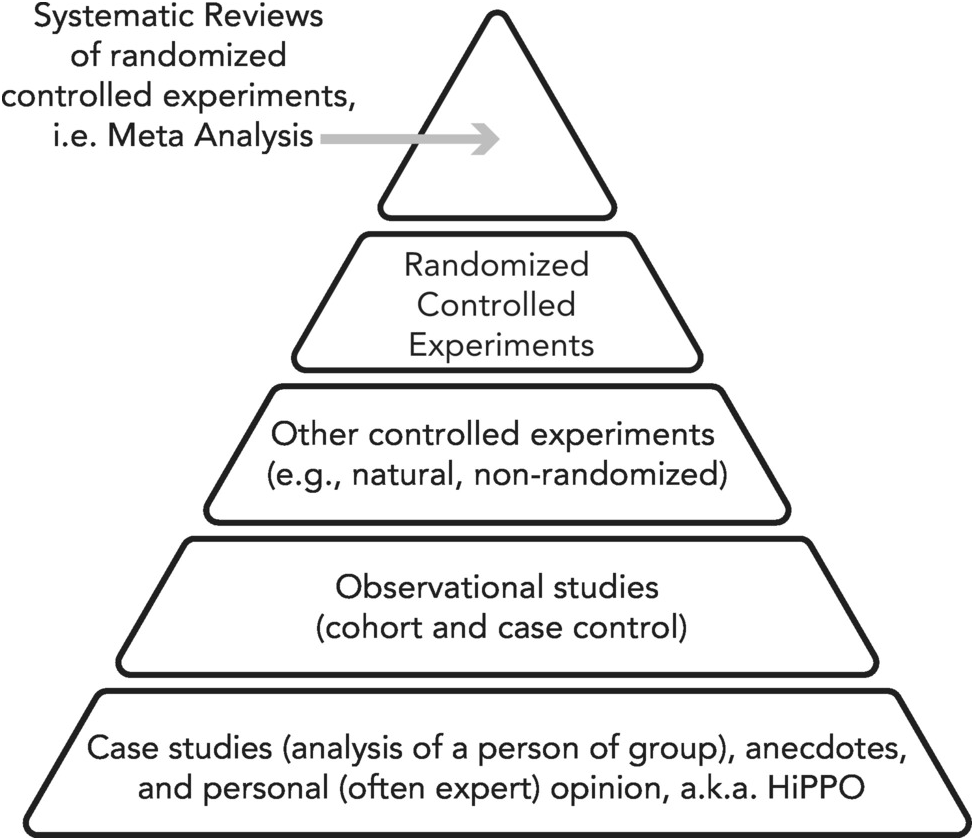
At the top of this pyramid, you have randomized controlled trials (RCTs) and meta-analyses.
These are the gold standard for establishing causality because they control for variables and aggregate results across multiple studies to find patterns.
Something I deeply miss from my career in growth and experimentation was the relatively cleanability to parse out elements in A/B tests and quantify their effects. While we can do this in some ways with SEO (A/B testing title tags, for example), there’s a lot more noise.
There are also non-randomized experiments we can lean on.
I’ll give you a counterintuitive example from our client portfolio.
We worked with an extremely large global brand with billions in revenue and the highest domain authority you could ask for.
One of the projects they asked us to help them with was to generate a large amount of high quality backlinks to a few key pages around generative AI.
I was skeptical, if not outright doubtful, about the impact of this engagement.
But they had budget, we had the resources and team in place, and their executives, for some odd reason, deeply cared about backlinks as a success metrics for their organic growth team.
So we took it on, driving ~80 links to 4 key pages from November 2023 to March 2024. It was an isolated effort – no technical pages, nothing on-page done, no new content or internal links, and it does seem like it moved the needle.
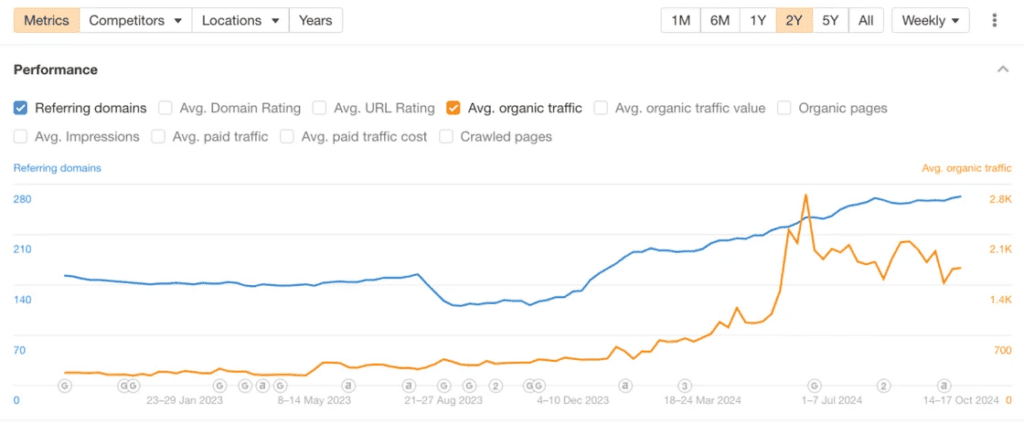
Again, this is non-randomized, so we have many underlying invisible variables that could have impacted this (user behavior signals, non-SEO campaigns, or merely time itself). But it’s a decent if not relatively weak signal that links matter, even at the highest peaks of brand and domain authority.
Next are observational studies, which, while not as robust as RCTs, help identify correlations by examining real-world data. In SEO, this might be the equivalent of analyzing broad industry trends to understand how tactics like backlinking, site structure, or keyword placement influence rankings. Luckily our industry is passionate about collecting this sort of industry data and sharing patterns with the rest of us (e.g. Lily Ray, Kevin Indig, etc.)
Lower on the pyramid are case studies and anecdotal evidence. These might include single-site SEO experiments or anecdotal reports from industry professionals. While valuable for generating hypotheses, these forms of evidence aren’t enough to bet the farm on. Just like in nutrition science, where a single success story about a diet might be inspiring, it doesn’t mean the results are replicable for everyone.
For instance, my hyperscaling of affiliate-based content on my personal website resulting in a big crash is an interesting data point. But it’s much more interesting when we can detect patterns from many sites who have done the same thing (which it does appear is starting to emerge with regards to mass-generated unhelpful content).
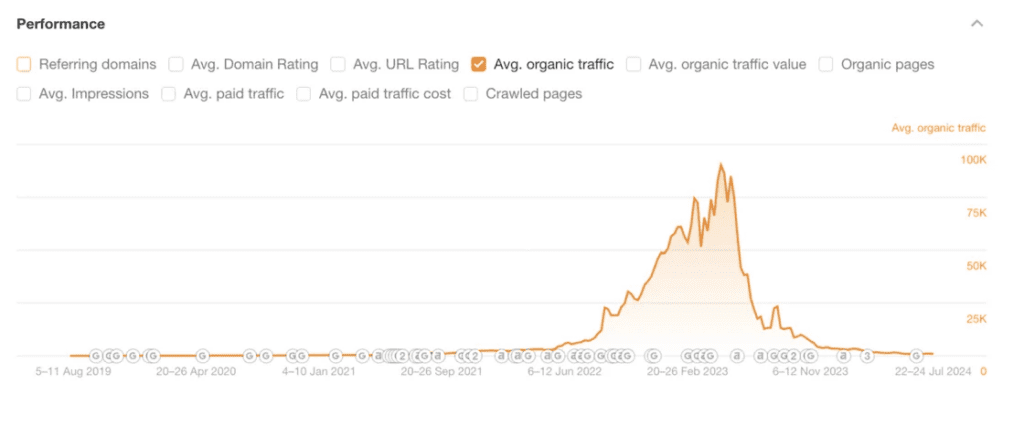
The key takeaway: The stronger the evidence, the more confidently you can move forward with your strategy. Weaker forms of evidence can be useful for guiding hypotheses and decisions, but you should weigh them against the risk and potential upside.
For instance, while case studies and anecdotal reports about link-building strategies might point in a certain direction, they need to be backed up by more reliable data before you pour resources into them.
Do no harm bets and what’s worth risking
When operating in a complex system like SEO, the goal is often to balance risk and reward. If you’re unsure about the value of a specific action, ask yourself two key questions: 1) How strong is the evidence? and 2) What’s the downside risk if the action doesn’t pan out?
Take something like a coldplunge in health and wellness. While there isn’t conclusive evidence that it offers huge benefits, the downside is minimal and northern Europeans have been doing this forever, so the “do no harm” principle suggests it’s worth trying if you’re interested. But if you don’t like the feeling of an ice bath, you don’t need to feel any FOMO just because every health influencer posts selfies doing them.
The same can be applied to SEO strategies. Testing creative copywriting in your title tags may not yield dramatic results, but it also won’t “harm” your site in any meaningful way. It’s also an easily reversible decision – a two-way door. It’s a low-risk, medium-reward scenario that’s worth experimenting with.
On the other hand, let’s consider more risky bets, like buying cheap backlinks from a questionable source. Sure, there’s anecdotal evidence that buying links can lead to short-term boosts in rankings, but there’s also significant downside risk—a potential sitewide penalty from Google.
Here, the weak evidence combined with the high downside means this is a risky bet you’re better off avoiding.
In our running nutrition science analogy, all too often I see health influencers making potential risky suggestions with little likely upside.
That’s been my contention with Andrew Huberman, who has recommended taking Fadogia Agrestis (which has been linked to testicular toxicity) and Tongkat Ali, which appears to activates Luteinizing Hormones (LH) that stimulates the Leydig cells to produce testosterone.
However, the few human studies available have shown these to be, for the most part, clinically meaningless. Not only could they have potential side effects (always possible when you mess with complex systems like hormones), but quality control for these supplements is notoriously terrible, so you have no idea the quality of the supplement you’re taking.
So little tangible upside, with mostly downside potential. Count me out. Not a risk vs reward tradeoff that I like. I’ll stick with diet and exercise and the occasional sauna.
In essence, “do no harm” bets are those where the upside is uncertain, but the downside is limited. These are often worth pursuing in SEO because the potential reward outweighs the minimal risk. Conversely, when you encounter tactics with weak evidence and substantial risks, it’s often best to steer clear.
Utility versus precision tradeoffs
One of the biggest traps in SEO—and in any complex system—is trying to achieve perfect precision in your models.
But here’s the truth: All models are approximations of reality. The point isn’t to model every single factor with 100% accuracy; the point is to make the model useful enough to guide your decisions.
For example, in SEO, we know, pretty broadly, that “links matter.”
But do we need to know the exact causal value of every single backlink? Not really.
It’s more productive to establish key principles based on the available evidence—things like the importance of relevant topic matter, the strength of a site’s domain rating, and the traffic to the linked page.
Notably, Google is likely looking at signals that are “hard to fake” or game, meaning it’s unlikely driving a ton of unnatural backlinks will have a large impact without associated brand equity and “organic” link acquisition.
They’re also likely more useful to establish baseline credibility and have decreasing marginal utility after you pass a certain threshold effect.
These broad strokes – a mixture of mechanistic knowledge with natural experiments and observational studies and a little bit of critical thinking – give us a good enough model to make informed decisions without getting bogged down in minutiae.
Chasing too much precision can actually reduce the utility of your model. The time and resources spent trying to measure the precise impact of every single link would be better spent executing strategies based on well-established principles.
In this sense, the cost of increasing precision can lower the expected value of your model. The goal should be to achieve a balance between accuracy and practicality—enough accuracy to be confident in your decisions, but not so much that you get lost in unnecessary details.
Not to digress too far, but that’s what frustrates me the most about the never-ending attribution modeling debate. It is both obvious and well-known that attribution models are flawed to some extent, but that doesn’t mean they are useless. It simply means you must acknowledge you’ll never have 100% precision and move forward with a model that is both flawed and useful in improving decisions.
In short, you don’t need to model every single element of a system to make it work for you. Use first principles like “links matter” or “content should solve searcher problems” or “our content should map back to our core product” and then move forward.
Perfect precision isn’t necessary for effective decision-making—what matters is having a model that’s useful for driving results.
Building a risk-adjusted portfolio of bets
We’re always operating under conditions of uncertainty, and while we have strong evidence of the efficacy of some variables, there are many variables we’re likely not even aware of. That shouldn’t prevent us from taking action and driving outcomes.
This is where I advise companies to establish a well-balanced portfolio of bets, and put more weight behind the actions you have the most confidence in. We use the “barbell strategy,” with a high portion of bets being safe and predictable and a small portion being lower evidence and higher potential upside.
SEO is like investing: some actions have a higher expected return than others, but the risk is always there. You can never be sure of the exact causal impact, but you can model your decisions based on the highest confidence predictions.
Here’s a good rule of thumb: if something clearly leads to an outcome—like publishing content clearly leading to traffic—it’s a high-confidence bet and more easily modelable. Without content, there’s no traffic, just purely from a logical perspective.
For other actions, like technical SEO changes or backlink strategies, you may have to triangulate industry patterns and observational data, mechanistic understanding of search engines and retrieval, and your own critical thinking to identify the top 5% of actions that will move the needle, ignoring the rest, as you build a high output organic growth system.
Want more insights like this? Subscribe to our Field Notes.